Findings from a poll of K-12 teachers, students, parents, and college undergraduates show that artificial intelligence (AI) has become intertwined with our education system. Increasingly, teachers, parents, and students are aware of and regularly use Generative AI tools (GenAI) such as chatbots.
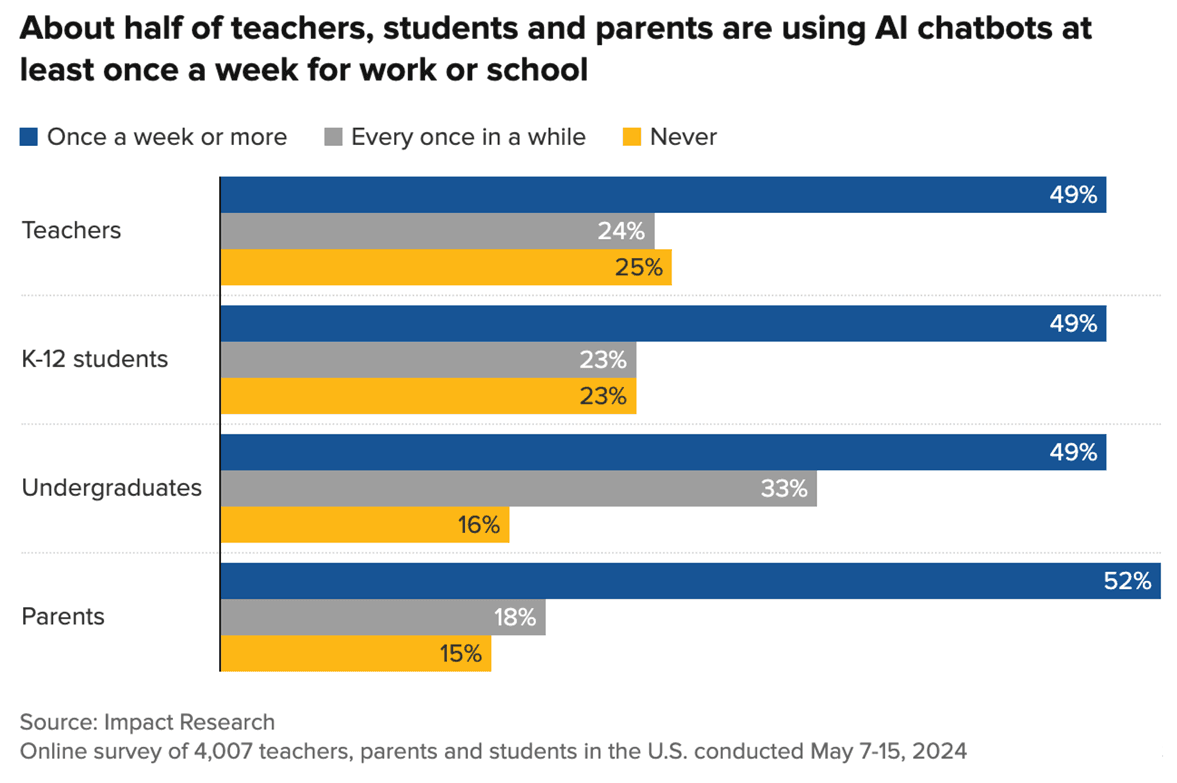
However, despite widespread use, most K-12 teachers, parents, and students don’t think their school is doing much about AI. Most say their school has no policy, is doing nothing to offer desired teacher training, and isn’t meeting the demand of students who’d grow to work in a world that will need AI skills.
Integrating AI into education will not be easy. Though teachers have endured a COVID-induced crash course in education technology, many are still behind the learning curve.
Only a handful of teacher training programs are moving quickly enough to equip K-12 teachers with a grasp of AI fundamentals—fewer still are helping future teachers grapple with more significant issues of ethics and what students need to know to thrive in an economy dominated by technology.
Teachers have a unique opportunity to take the lead in integrating AI into classrooms. By proactively seeking out resources, experimenting with AI tools, and focusing on practical applications, teachers can begin to fill the void left by slow-moving policies.
In this article, we will explore some tips for achieving immediate results, as well as long-term strategies that will require school-level support. The goal is to transform AI from an abstract concept into a valuable classroom ally, driving engagement and learning outcomes.
Five Strategies to Unlock Immediate Benefits with AI
Realizing the full potential of GenAI will require thoughtful planning and coordination, both at the teacher and school levels. It may also call for updates to classroom-support software (such as LMS) to integrate some of the features. However, to effectively use AI in the short term, teachers can apply the following five evidence-based strategies in the K-12 classroom:
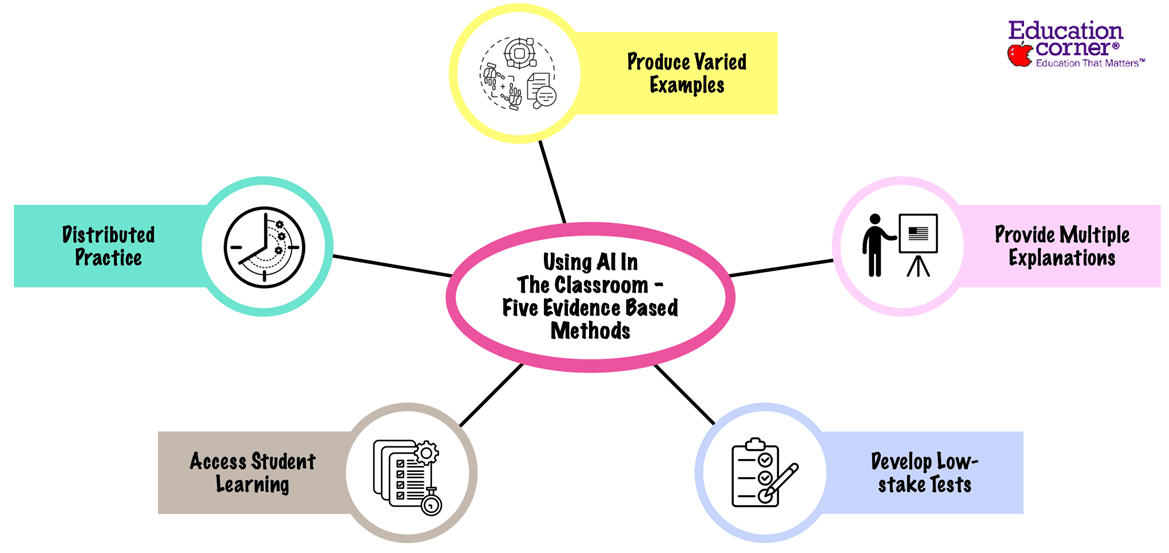
Use AI to Produce Varied Examples
Adding many varied examples helps students better understand complicated concepts and ideas. It also helps decontextualize ideas from examples, leading to better recall and understanding. While this process is time-consuming, it can be effectively outsourced to AI.
Consider the examples ChatGPT generated for the prompt:
“I would like you to act as an example generator for fifth-grade students. When confronted with new and complex concepts, adding many and varied examples helps students better understand those concepts. I would like you to provide me with ten different, varied, and accurate examples of applying fractions to everyday problems around us.”
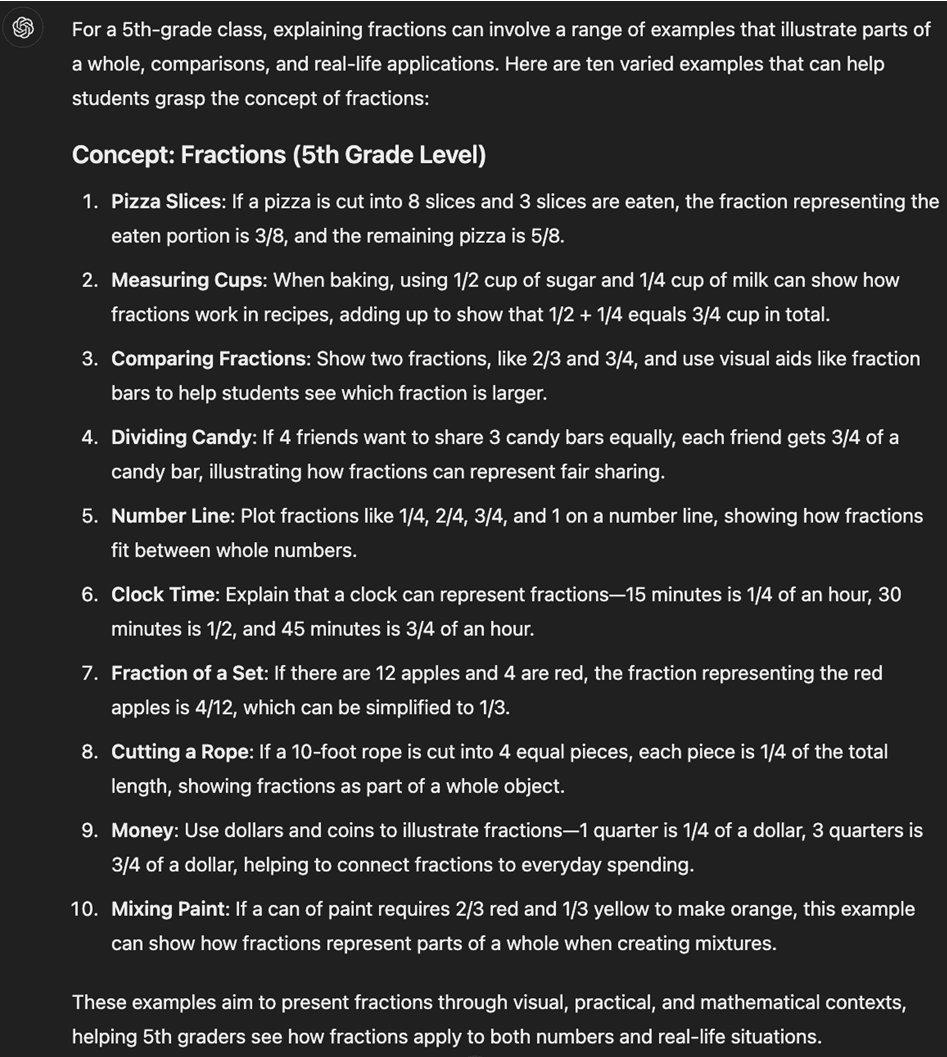
Different LLMs can generate different outputs and a variety of examples. Teachers can experiment with different models to learn which works better for their prompts. Access the output carefully by asking the following questions:
- Are the examples relevant?
- Are they factually correct? AI examples can be subtly wrong at times.
- Do the examples have enough detail?
- Will they interest students?
- Are they varied? Do they approach the concept from various perspectives?
- Do they serve to connect the abstract (concept) to the concrete (real-life application)?
Upon evaluation, AI’s output can be deployed in the classroom in a number of ways – weave them into a lecture, post them as additional notes or material for a lesson, or use them to give students additional practice.
If students have a knowledge base about the topic, you can even use a wrong or subtly wrong output as an advanced exercise: “Which of these examples demonstrate concept X? Which do not? Explain your reasoning.”
Use AI to Provide Multiple Explanations
Effective explanations lay the groundwork for foundational knowledge that helps students build mental maps of topics. However, producing many explanations is complicated and time-consuming for various reasons:
- Student knowledge can vary across subjects.
- Concepts can be abstract or wholly unfamiliar.
- Students may need multiple explanations adapted to their level of understanding, including examples, models, and demonstrations.
- Explanations may need a lot of background information that students may not have.
This is where AI can help adapt explanations to the demands of student learning levels by generating multiple explanations from various perspectives—explanations that use a step-by-step approach or add details to any existing explanations.
Ensure you use AI-generated explanations as a starting point and vet them before they reach students. Use the following process to ensure the best results:
- Start by picking a concept you want to explain.
- If using an AI chatbot connected to the internet (such as Bing), prompt the AI to look up the concept, using core works in the field.
- Instruct the AI on what you are looking for (a clear multi-paragraph topic explanation).
- Describe your writing style (clear, straightforward, concrete, dynamic, engaging).
- Describe your audience.
- Follow up the initial prompt by asking for analogies.
An example prompt could be as follows:
“Look up the concept of Photosynthesis using core works in plant biology and botany. Provide a clear multi-paragraph explanation of photosynthesis, detailing how plants convert sunlight, water, and carbon dioxide into glucose and oxygen. Write in a clear, straightforward, and engaging style.
Audience: My students are fourth graders encountering this concept for the first time, so keep the explanation simple and avoid overly technical language.
After the initial explanation, provide analogies that relate photosynthesis to everyday processes, such as how a plant’s chlorophyll is like a solar panel converting sunlight into energy.”
The next step is to assess the explanation using the following questions:
- Is the explanation clear and consistent?
- Does it use language that is unambiguous and easy to understand?
- Does it focus on the most critical parts of the topic(s)?
- Is the explanation factually correct?
- Is the explanation adapted to student learning levels? (work with AI to simplify if required).
- Is the explanation coherent and engaging, and will it be helpful to students?
- Does the explanation connect to students’ prior knowledge about a topic? (ask to include specific ideas or make connections to previous topics).
You can even include these explanations along with course materials, augment any demonstration with an extended explanation, or post it as a study guide.
Use AI to Develop Low-Stakes Tests
Frequent, low-stakes tests are effective not only for assessing knowledge but also as learning experiences. Regular testing and knowledge retrieval aid in long-term information retention. They also provide students with timely feedback, allowing them to focus on closing gaps in their knowledge and adjusting their learning strategies.
Developing quizzes, multiple-choice or short-answer tests, or intentionally adding questions that test knowledge within lectures requires effort. Teachers may not have enough time to develop varied and frequent tests and may not have a reliable way of grading such tests, especially if they include short answer components. Tests must also be designed so that they are neither too easy nor too challenging.
AI can help teachers generate practice tests, quizzes, and short answer tests about a topic or a reading and can help add questions that test student knowledge within lectures. Use the following steps for best results:
- Let the AI know its role. In this case, a creator of diagnostic quizzes.
- Inform the AI of the topic you want to teach and the teaching strategy you want to pursue (low-stakes test to assess student knowledge).
- If you have used a publicly available document or article in your teaching, share the article(s)/URLs in the prompt.
- Give directions about the types of multiple-choice questions you expect. For instance, multiple-choice questions should include plausible, competitive alternate responses and not include an “all of the above option.”
- Check its output; you may need to work with it to develop factual and higher-order questions.
For any test the AI generates, evaluate the output and make sure it is accurate and adapted to the right level.
Multiple-choice tests must be carefully worded to include plausible alternatives to the correct response. These alternatives can be challenging to generate because they should be attractive to students with incomplete understanding and based on common misconceptions students have about the topic.
Similarly, short-answer questions must be straightforward, unambiguous, and not open to interpretation. Also, ensure that any AI-generated tests align with the unit or course goals so that success indicates command over the topic.
Using AI to Assess Student Learning
There are hundreds of variations of classroom assessment techniques that can help teachers and students monitor their learning and understanding. It is effective because such techniques provide immediate feedback about what students know and, crucially, what they are confused about.
Most begin by asking students to summarize and interrogate their knowledge and identify areas of confusion. Some typical questions include:
- What was the most important idea or concept covered in class today?
- Why do you think this idea is important?
- What is the most challenging concept so far?
- What did you struggle to understand?
- What concept or problem would you like to explore in more detail?
While teaching moments can be identified within such responses, consistently doing so is often challenging due to a lack of time to review every response. Teachers might also struggle to focus on specific answers or interpret them in ways that align with their perspectives.
Because AI doesn’t know your class, it can provide a balanced view of student responses and can be used to summarize responses quickly. Teachers can create a shared document or form and ask students to submit their responses. A collective set of responses can then be fed into the AI to glean insights. Use a prompt such as:
“I am a teacher who wants to understand what students find most important about my class and what they are confused by. Review these responses to identify common themes and patterns in student responses. Summarize responses and list the three key points students found most important about the class and three areas of confusion {insert URL or share text response}.”
Teachers can continue questioning the output, asking AI to help explain points of
confusion and develop explanations. They can also adjust teaching in the following classes to clarify misconceptions, provide additional resources, or address student gaps.
To evaluate the AI output, check the following:
- Do these align with your teaching goals for the class?
- Can most students explicitly name and explain the key ideas? Verify the common points of confusion.
- Have these typical gaps been seen before?
- Verify student responses if the output does not match your expectations or if you are surprised by what you uncover.
When students are pushed to name key ideas and identify points of confusion explicitly, they may worry about revealing their struggles. An anonymous survey or discussion board works best; the latter reassures students they are not alone in their struggles.
Such assessments can also be dynamically conducted mid-class. Have students post the responses, upload them to the AI, and share the output to discuss the results:
- What did the AI point out?
- What patterns did AI highlight?
- What common areas of confusion do students hold?
This can lead to conversations where students answer each other’s questions and clear confusion through a facilitated discussion.
Use AI in Distribute Practice of Important Ideas
Students stay up late into the night, cramming weeks of material into one study session before the final exam, only to forget the material as soon as the exam is over. Distributed practice, also known as spaced repetition or spaced practice, can address this challenge.
This learning strategy divides the practice into several short sessions over a longer period, helping students make better connections and improve information retrieval. However, the challenge in implementation is that most course materials are simply not designed to align with this practice.
They are constructed for mass practice–one topic after another, in a linear fashion, without reference to the previous, often lacking any direct connection between each other.
To include distributed practice in a class requires that teachers assess the following:
- What are the most important course topics?
- Which connections between topics are critical and must be practiced often?
- How and when should students practice making connections between topics to retrieve previously learned information?
Doing this while also introducing new topics can be time-consuming and challenging. GenAI can make this process easier.
One way to include distributed practice in a course is to introduce a topic and review it after a week, a month, and then at the end of the semester. Teachers can direct AI to generate brief topic overviews and questions that test student knowledge and then use those as part of ongoing assignments or assessments at predetermined intervals.
If most students respond correctly, AI can dynamically increase the difficulty level of the questions or alternate the question sequence.
AI can also weave previously taught topics into ongoing lectures or discussions to test students. Ask AI to incorporate ideas or facts into a current topic, find connections, and help make those connections explicit to students. An example prompt could be:
“You are an expert teacher who provides help with the concept of distributed practice. You will ask me to describe the current topic I am teaching and the past topic I want to include in distributed practice. You will also ask me about the audience or grade level for the class. Then, you will provide four ideas for including the past topics in my current topic. You will also provide two questions I can ask the class to refresh their memory on the past topic.”
Make sure you check the output on the following points:
- Facts and consistency – some connections may not be critical.
- Are the suggested exercises and tests related to key topics?
- Will they help students connect new and old information?
- Are they suitable given the prior knowledge and the time frame?
- Are they realistic and manageable from a teaching perspective?
- How about variety and creativity–are they repetitive, and do they offer different ways of reviewing the topics?
While these were the five ways to use AI in the short term, we will now look at some long-term roles AI can play in assisting teachers and students in improving learning outcomes.
Using AI In The Long Run: Student-Supporting Roles
Adaptive Learning
Adaptive learning environments use technology to modify the presentation of educational material in response to each learner’s needs. They use a combination of technological and classroom-based systems to tailor learning experiences based on factors like pace, modality, path, and learning preferences.
Based on the degree of involvement, AI-based adaptive education can be ranked into six levels (L0-L5) as shown:
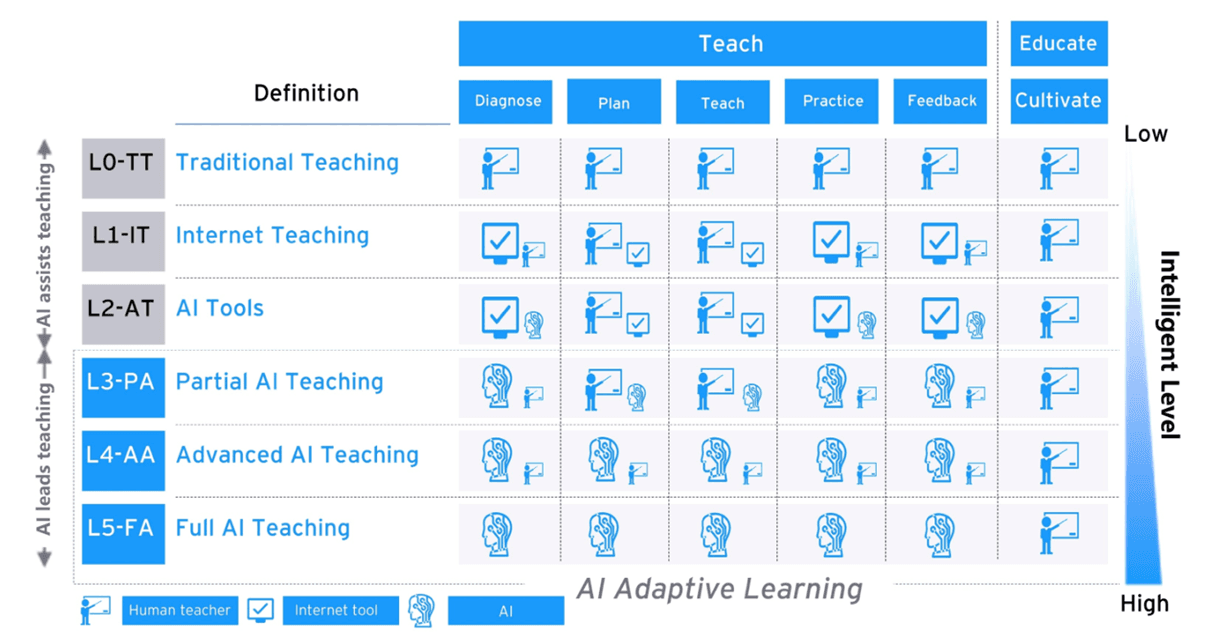
At an advanced level, an AI tutor will know the material well enough to provide personalized, adaptive learning exercises based on student’s needs and abilities. However, this will require the complete ecosystem of supporting software, such as LMS, to evolve.
AI companies like OpenAI have invested billions of dollars into developing foundational models that are becoming increasingly capable. Once developed, the marginal cost of deploying these models is minimal, making it feasible to scale powerful GenAI-driven adaptive learning applications.
Such platforms can integrate tools for image creation, data visualization, tutoring, coding, knowledge management, and more to facilitate large-scale personalized learning, aligning with the principle of “teaching according to one’s abilities.” This can aid adaptive education in several ways:
Profile Building
Learner modeling involves creating and maintaining individual learner profiles and collecting data such as assessment scores, learning preferences, progress tracking, and even socio-emotional factors.
AI algorithms can analyze large amounts of data to make intelligent predictions about learner needs, preferences, and future performance by identifying patterns, trends, and relationships. This enables adaptive learning systems to dynamically adapt content, pacing, and teaching strategies to individual learner needs, facilitating a personalized and streamlined learning experience.
Material recommendation and content customization
Most computer-based adaptive learning methods available today use one of the two approaches:
First is a set of pre-established rules to guide learning. For example, “if the learner achieves 60% in module-1, module-2 is unlocked; otherwise, a revision module is offered.” While less efficient, this is easy to implement and requires fewer resources. However, it demands detailed educational design, as educators must define mastery scores, learning paths, and multiple branches, which can be time-consuming and require specialized expertise.
The second approach leverages advanced machine learning, such as deep learning, applied to large learner cohorts. It uses dynamic models that update with new learner data, like exercise answers, to create detailed skill representations and suggest optimal content for progress.
While this level of personalization yields superior results, it requires extensive data for accurate modeling. Additionally, content created with different authoring tools in an LMS often produces varied results, necessitating standardization before these algorithms can effectively adapt learning experiences.
GenAI opens a third approach by combining the simplicity of the first with the power of the second. Because it can generate content, it can create specific content on the fly and tailor it to each learner’s individual needs. A first step in this direction is already visible in the form of chatbots on e-learning platforms. Learners are no longer left alone: they ask questions to a chatbot powered by GenAI that responds based on specific needs.
Intelligent agents
An AI agent or an intelligent agent is a software entity that perceives its environment and takes action to achieve specific goals. These agents operate autonomously, making decisions based on data and predefined programmed algorithms. AI-powered agents provide options to help make online learning cost-effective while increasing learning gains.
For example, the team working on Project RAISE, a collaborative project between UCP of Central Florida and the University of Central Florida, has developed a socially assistive AI companion called Zoobee. The AI agent supports students with autism spectrum disorder (ASD) in learning coding, science, engineering, and mathematics (STEM) content while developing their social-emotional communication skills.
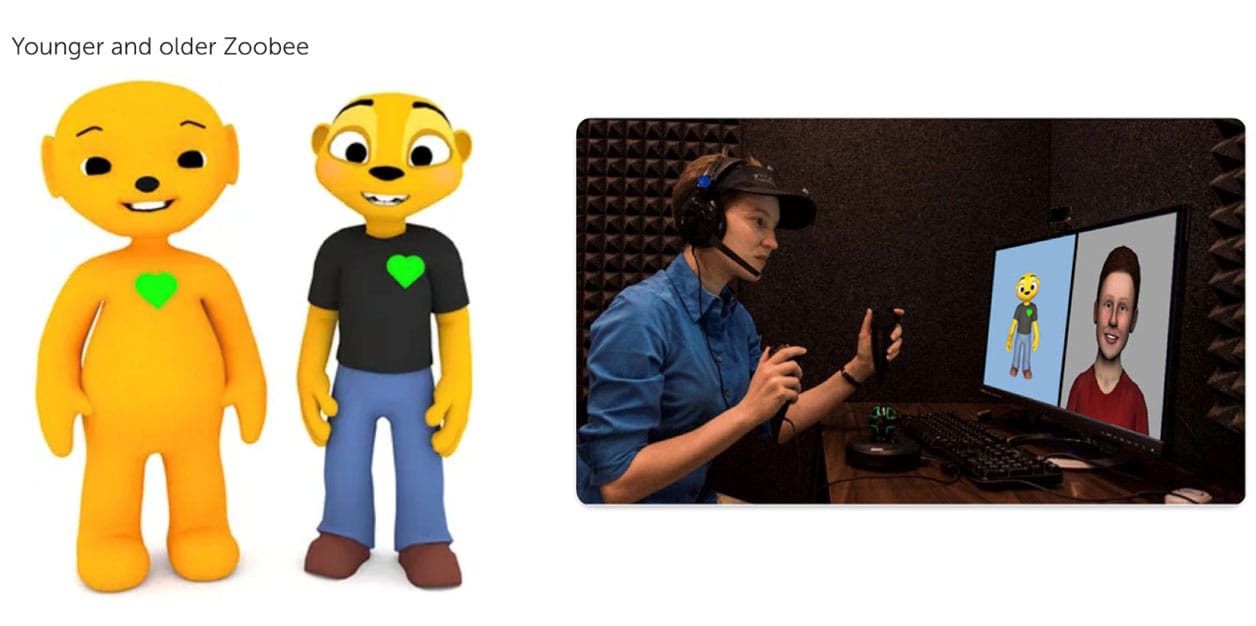
Zoobee was initially created as one character, but the need for older and younger versions emerged as the project progressed. The AI agent currently exists in two varieties: the first is designed for children in the early elementary grades (Pre-K – Grade 2), and the second is designed for upper elementary students (Grades 3–5).
The latter version was co-designed by students, including those with ASD, who voted on several character variations. This process of student-driven AI development makes the agent relatable and acceptable to the population targeted for use.
While Zoobee did not use GenAI, today, advancements in natural language processing and GenAI allow such AI agents to become more expressive and capable. They can respond better, given the advances in multimodal data collection, recognition algorithms, and signal processing.
In Australia, Cogniti, an early-stage pilot GenAI platform built by a team from the University of Sydney, allows teachers to create “AI doubles” of themselves. These agents help students and teachers in various settings, including:
- Helping to understand fundamental concepts in chemistry.
- As a helper for group work projects.
- Help improve writing skills by providing feedback on practice assessments.
- As a Goal setting agent.
- As a classroom simulator to help tutors improve their teaching skills.
- As a study buddy, providing advice based on learning theories to bust myths about learning.
Khanmigo—produced by Khan Academy, an education non-profit powered by ChatGPT—can do many other startling things. Ask it a math problem, and it will guide you through the steps required to figure out the answer for yourself. Ask for help writing a science-fiction story, and it will trade you a sentence for a sentence until your hero gets gobbled by an alien.
These examples are just a glimpse of what can be possible in education using AI-powered intelligent agents.
Learning Simulations
Although the effectiveness of simulations in enhancing learning outcomes is proven, their widespread adoption remains challenging. They are difficult and expensive to create, require detailed scripting and lesson design, and must be performed by designers and developers working with subject matter experts.
Creating multiple outcomes and giving learners control over decisions requires pre-planned design and programming. There is no single unified simulation platform, and they require expensive customized software and additional changes or adaptations for particular audiences or classroom contexts.
Tracking student performance is also challenging, as combinations of choices and complex interacting factors make understanding individual pathways through simulated environments difficult. As a result, simulations are underutilized despite their immense potential.
GenAI allows for the broader deployment of high-quality teaching simulations by leveraging a system of multiple AI agents to provide personalized learning experiences. Students can practice skills with AI-generated mentors, role-players, and instructor-facing evaluators.
For example, the Generative AI Lab at Wharton has developed two agent-based simulations, one for K12 teacher training and one for entrepreneurial pitching. Here is how their approach changed with GenAI:
Prior to the use of Generative AI | The AI Now Plays the Role of |
---|---|
Video instruction with Q&A follow-up. Instruction material was general, with follow up “mentor” conversation limited to prewritten questions with limited choices. | A Mentor Agent – Responds to questions about the topic. – Adapts to the student’s level of experience. – Tailors conversations to student open-ended responses. |
In-game scripted characters or Non-Person Characters(NPCs): Interacted with students via predefined responses providing limited reactivity. Character development took considerable time and effort. | With AI Agents: – Have a backstory. – Respond based on the student’s performance. – Can improvise and adapt to any variety of student responses. |
Branching storylines: Limited in scope and pre-crafted to cover limited choices. | AI Game Master Agent: – Evolves the storyline based on the student’s actions. – Each playthrough can be different as the AI adapts responses. |
Feedback: Provided based on student-tracked choices that are hard-coded into the system. | AI Coach/Advisor: – Gives tailored feedback after the simulation. – Bases feedback on specific student performance and lesson elements. |
Class insights report for instructors: Based on student choices with insights into specific decisions students made. | Class Insights Agent: – Analyzes all interactions between students and AI NPCs. – Provides assessments of individual and class performance. – Identifies gaps and common errors. |
Suggestions for debriefing and class activities: Static debriefing materials for discussing the simulation and follow-up activities. | Instructional Agent: – Reads Class Insights reports. – Provides targeted suggestions for debriefing and follow-up activities based on class performance. – Helps tailor activities to address gaps in student knowledge. |
What does this mean for simulation-based teaching in K-12? Let’s explore with an example:
In traditional K-12 simulation-based teaching, students might watch a pre-recorded video about a topic like the solar system. After the video, they are prompted to answer multiple-choice or true/false questions based on the content.
A question might be: “What is the largest planet in our solar system?” The student selects from preset answers, such as a) Jupiter, b) Earth, and c) Saturn. If the student selects the wrong answer, the system provides a generic response like, “Try again!”. This process offers limited interaction and is mostly one-size-fits-all, unable to adapt to a student’s unique understanding or queries.
With GenAI, the instructional approach shifts to a more personalized experience:
- AI responds to questions about the topic: After a lesson on the solar system, a student might ask, “Why does Jupiter have such a strong magnetic field?” The AI mentor agent can provide a nuanced explanation tailored to the student’s query, diving into the science of planetary magnetic fields and relating it to Jupiter’s size and composition.
- AI adapts to the student’s experience level: If a younger student asks, “Why is Jupiter so big?” the AI mentor might explain in simple terms, using analogies like, “Think of Jupiter like a giant balloon filled with gas!” For an older student with more knowledge, it might delve into the planet’s formation process in the early solar system.
- AI tailors conversations to students’ open-ended responses: When a student says, “I’m interested in how planets form,” the AI can engage in a deeper, unscripted discussion about planetary formation, including topics like protoplanetary disks, gravity, and accretion. It might even suggest topics like exoplanets or encourage the student to explore how other stars form their planetary systems.
This evolution from a static Q&A system to a dynamic AI mentor enables a more interactive, adaptive, and personalized learning experience.
Role Of AI in Boosting Creativity
Education systems have always struggled to fully support creativity. Unique ideas and approaches are antithetical to multiple-choice standardized assessments, where there is one “right” answer. Textbook-based curricula and lesson plans focus on optimizing content knowledge and operations rather than asking students to engage with ideas and connect in various ways.
Teachers across disciplines and grade levels have always desired to teach more creativity. GenAI can remove some of these structural barriers to support research-informed pedagogy that amplifies students’ creative thinking skills and helps personalize learning through the creative process. With proper scaffolding and human oversight, GenAI tools can potentially support students in open-ended creative tasks.
AI supports students’ divergent thinking. For instance, in a K-12 classroom, students could begin by choosing an issue that interests them or they are passionate about. One student may use GenAI to ask questions and better understand a problem, another may take help in brainstorming different ways of visualizing an idea, and a third student may decide to use AI for assistance in evaluating and deducing which of their ideas is most effective, then articulating and sharing their thought process.
Teachers, however, must be mindful of some of the risks associated with this approach. When kids see AI as something to learn from and that their [AI] tutor knows everything, they may feel they can never be as smart as it. They could lose confidence in their intelligence. Children must think of AI as a collaborator—what can the tool do, and what does it bring in? They must have the voice and authority to give feedback.
“When kids see AI as something to learn from and that their [AI] tutor knows everything, they may feel they can never be as smart as it. They could lose confidence in their own intelligence.”
A key feature of using GenAI to boost creativity is seeing it as a tool that can augment the students’ creative process, not replace it. It must be used to support their brainstorming and produce design options far faster than they could, giving them more choices and highlighting their role in discerning which is most effective and why.
GenAI helps students articulate their knowledge and skills with text, images, and other media. They could share the various iterations they went through, with or without AI, to explain the effort and different directions they took before landing on the outcome.
GenAI tools alone may or may not help kids learn. The essential piece is a “human in the loop” approach where learning is supported by teacher oversight through thoughtful pedagogy and intentional learning design.
Using AI In The Long Run: Teacher-Supporting Roles
A good next-generation technology should adapt to teachers’ style and approach, and GenAI can do that. Due to this, it can support teachers in various ways:
Lesson Planning And Content Differentiation
Traditional education, with a single teacher managing dozens of students, often relied on a one-size-fits-all approach due to time constraints and the challenge of tailoring the learning material and lesson plans to suit diverse needs at scale.
While the advantages of differentiated instruction are widely recognized, its implementation remains difficult. The primary hurdle is delivering varied learning experiences to a classroom of 20+ students, which can drive teachers back to uniform teaching methods.
Studies show that teachers with classes of 30 or so students inevitably end up spending much of their time delivering material at a speed and level of difficulty that suits an average child. Some children master the material effortlessly and are at risk of getting bored. Others need a lot of help; a number of them will end up falling behind.
GenAI models offer a powerful solution, swiftly generating lesson plans and learning materials based on specific prompts. For instance, with GenAI, a lesson on “How Plants Grow” can be easily tailored to accommodate the varied learning preferences of a diverse 2nd-grade class. The below example was generated using OpenAI’s ChatGPT using the prompt shown:
Prompt: “I’d like to create a lesson plan to teach my 2nd grade students on how plants grow. However, I have a wide range of learners in my classroom, some like to read, some like to learn through play and some like to listen to a story. Can you please provide a differentiated lesson plan that will help serve both audiences?” |
Lesson Title: How Plants Grow |
Grade Level: 2nd Grade |
Duration: 60 minutes |
Objective: Students will understand the life cycle of a plant and identify the basic needs of plants (sunlight, water, air, soil). |
Materials: |
– Picture books about plants (e.g., From Seed to Plant by Gail Gibbons) |
– Story cards with images of plant life stages (seed, sprout, plant, flower, etc.) |
– Planting materials: seeds, soil, small pots, watering cans |
– Growth chart or observation journals |
– Drawing supplies (paper, crayons, markers) |
Lesson Outline: |
#1. Introduction (10 minutes) |
– Whole Class: |
– Show a time-lapse video of a plant growing from a seed. |
– Discuss the different stages: seed, sprout, seedling, mature plant. |
– Ask students what they think a plant needs to grow. Write responses on the board (sunlight, water, air, soil). |
#2. Differentiated Activities (30 minutes) |
Group A: The Readers |
– Activity: Independent Reading & Worksheet |
– Provide picture books like From Seed to Plant by Gail Gibbons. |
– Students read about plant life cycles and complete a worksheet where they match pictures to the correct stage. |
– Extension: Write a few sentences describing each stage in their own words. |
Group B: The Play-Based Learners |
– Activity: Planting & Role-Play |
– Students plant seeds in small pots. |
– Engage them in a role-play activity where they act out being different parts of a plant (roots, stem, leaves). |
– After planting, they water their seeds and place them near sunlight. |
– Extension: Create a daily “care routine” chart for their plant, including watering and observing changes. |
Group C: The Story Lovers |
– Activity: Storytime & Discussion |
– Read aloud a storybook about a seed’s journey (e.g., The Tiny Seed by Eric Carle). |
– Use story cards to sequence the life cycle stages after reading. |
– Discuss about what the seed experiences at each stage, focusing on how it “feels” when as it grows. |
– Extension: Draw a picture of their favorite stage of the plant’s growth and write a sentence about it. |
#3. Wrap-Up & Sharing (10 minutes) |
– Whole Class: |
– Bring everyone together to share what they did. |
– Ask each group to share one new thing they learned about how plants grow. |
– Display their drawings, worksheets, and “care routine” charts on a bulletin board. |
#4. Observation & Reflection (10 minutes) |
– Individual: |
– Give each student an observation journal to record the changes they see in their planted seed over the next few weeks. |
– Ask students to draw and describe what they observe weekly. |
Assessment: |
– Participation in activities. |
– Completion of worksheets or drawings. |
– Ability to explain at least two things a plant needs to grow. |
– Observation journal entries for continued engagement. |
You’ll notice that this is a great start in that it covers all of the most important components.
Coaching And Feedback
Teachers and AI systems bring distinct yet complementary strengths to education. GenAI tools that process natural language can deliver real-time feedback on instructional practices, offering valuable insights into how teachers interact with students.
A substantial body of research supports the idea that teachers can improve their practice by reviewing their lessons. Previously, such analysis required manual recordings during coaching sessions, but AI now streamlines this process, analyzing live feeds and generating reports instantly. These tools are accessible from anywhere, enabling teachers to reflect beyond traditional coaching cycles.
LLMs powering tools like ChatGPT can transcribe audio files and extract valuable insights, helping teachers balance their speaking time with opportunities for students to engage. Research indicates that this balance is crucial for enhancing students’ learning experiences.
Edtech startups such as TeachFX provide AI-powered data reports that identify more than 20 different insights, such as whether teachers are building on what students say, whether they are asking open-ended questions to push student thinking, and how often they’re using academic language in lessons. The technology automatically analyzes the content of the files teachers upload, highlighting specific words and phrases.
Another AI Coach by Edthena guides teachers through self-observation and classroom action planning. It features automated interactivity that lets teachers design, implement, and iterate on their own professional goals via chatbot.
The data provided by AI tools can lead to better conversations, deeper reflections, and overall growth in teaching skills.
Grading And Assessments
Grading and assessments are another area of application. Because people and computers don’t always notice the same things in student writing, GenAI tools can provide a first layer of feedback before teachers evaluate assignments.
For example, CoGrader is an AI-powered Essay Grader that helps teachers provide quality feedback on essays in 80% less time. The app even accepts handwritten assignments, which can be uploaded as a scanned file.
Because the purpose of grading is not grading itself but providing feedback so students can learn faster, GenAI-based solutions can help teachers scaffold (not replace) the human element and make the grading process faster and more accurate.
Handling Limitations And Challenges
Inaccuracies and Hallucinations
By virtue of their style of writing and the way they impart information, GenAI models can appear to be trustworthy and authoritative. However, they can often produce inaccurate text. These factual errors are known as “hallucinations.”
AI hallucinations come in many different forms, ranging from funny contradictions to questionable facts to utterly fabricated information:
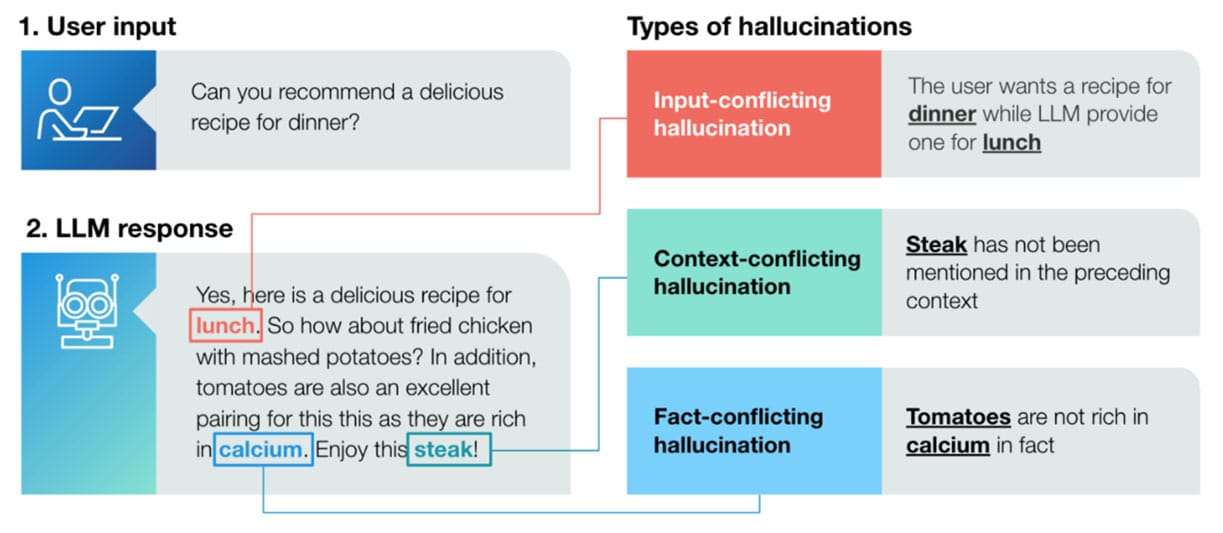
Input-conflicting hallucinations
These occur when an AI’s output does not align with specific inputs. This type of hallucination can manifest in various ways, such as responding to a request for information about a future event with data about the past or answering questions about one subject with information relevant to another.
For example, if a teacher asks for a math activity suitable for a 2nd-grade level and AI suggests science activities appropriate for high school students, this would be an input-conflicting hallucination. In educational settings, such hallucinations could result in lesson plans or activities that are mismatched to students’ learning levels, potentially hindering their understanding and progress.
Context-conflicting hallucinations
These occur when the AI’s response includes information or elements that are out of place, given the context of the query. This can include irrelevant or tangentially related information that does not address the user’s needs directly.
For example, in a K-12 setting, if a teacher asks for a relevant 4th-grade science experiment to demonstrate the concept of gravity, the AI may suggest an experiment about photosynthesis. Even though the suggestion might be scientifically accurate, it is irrelevant to the context of teaching gravity.
Fact-conflicting hallucinations
These involve the generation of factually incorrect content. These can be outright falsehoods or plausible but incorrect assertions that can mislead users or decision-makers.
For example, in a K-12 setting, an AI might generate a quiz on the water cycle that inaccurately states it involves only evaporation and precipitation, either neglecting condensation entirely or incorrectly marking it as a wrong answer. This can mislead students, weaken their understanding, and create additional work for teachers.
Dealing with Hallucinations
Using good-quality prompts can minimize hallucinations. Here is a five “S” framework to generate good prompts:
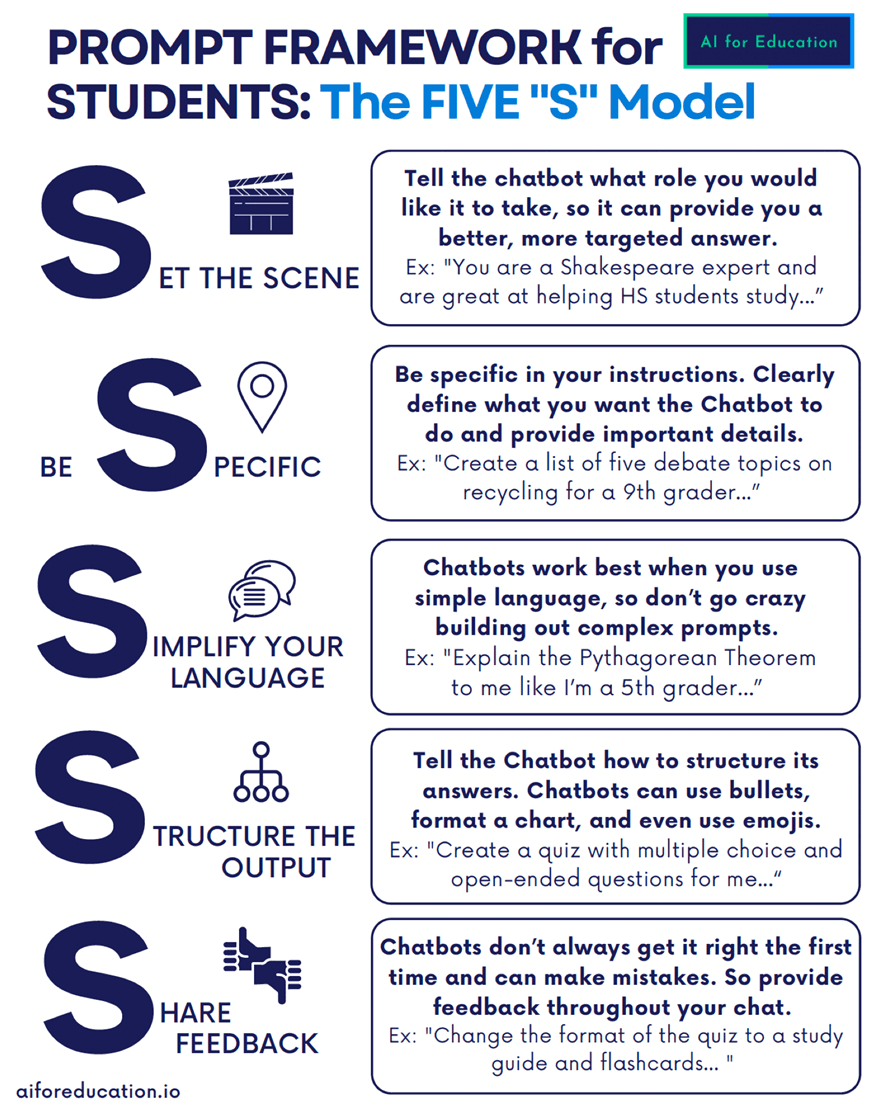
Teachers can limit the negative impact of AI hallucinations and misinformation by showing students examples of AI-generated output (text/images) using specific prompts and discussing the fallacies illustrated in the output.
Remind students to include requests for source links when using AI to research. (Some tools will supply links to their source material when requested.) Ask them to check each link and explain how AI can combine information from its data sets to generate complete fabrications.
Bias, Inequity, and Emotions
Just as GenAI can output incorrect information as fact, it can churn out bias as fact. Because AI can only learn from its source data, all the implicit biases in the original material—misinformation and problematic content—will be woven into the tool’s framework.
For example, ChatGPT, when asked to speak in the cadence of the author of a book featuring a Black protagonist, added the word “yo” in front of random sentences.
While AI companies are doing more to eliminate bias, educators must ensure that output doesn’t exhibit bias. Use media (movies, shows, and books) to introduce discussions about algorithmic bias, the flaws of facial recognition, and more.
AI tools can also claim to feel love, experience various human senses, and more. So students (especially younger ones) need to understand that an AI chatbot may sound like a real person, but it’s designed that way—by real people.
Cheating And Plagiarism
Ever since the release of ChatGPT, there has been significant debate surrounding the impact of text-based GenAI in education. Among various concerns being discussed, teachers worry the most about cheating and plagiarism:
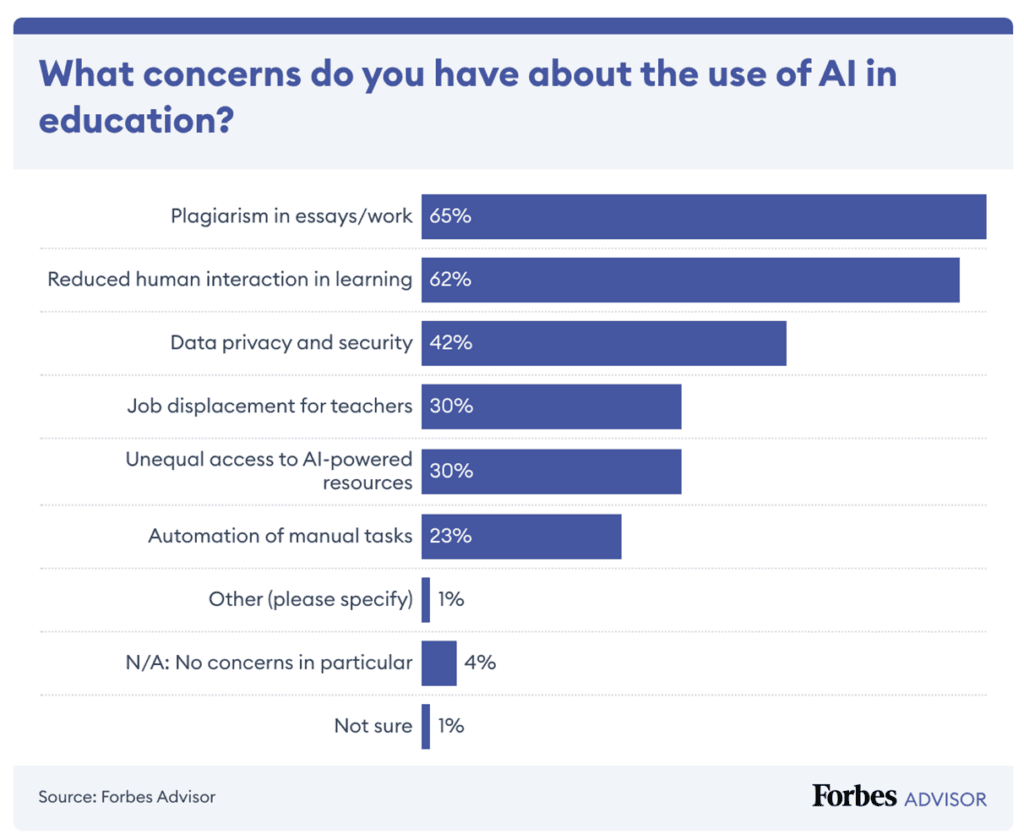
This concern is justified, for forensic psychology reveals that cheating often stems from a combination of motive, means, and opportunity. With AI, the opportunity and, particularly, the means to cheat have become omnipresent, while the risk and effort required to cheat have significantly decreased.
However, research also shows that many, if not most, students genuinely want to learn and avoid cheating (though a proportion of them will always look for shortcuts). This highlights the need for teachers to develop assessment strategies that address the underlying motives for cheating while preserving the benefits of AI tools for enhancing learning outcomes.
The University of Queensland’s Jason Lodge, along with Sarah Howard and Jaclyn Broadbent proposed a taxonomy of approaches to assessment redesign, which consists of six options as summarized:
What it means | Pros | Cons | |
---|---|---|---|
Ignore | Proponents believe GenAI is a hype and choose to ignore developments and hope it goes away. | No Effort needed | Unlikely to be viable long-term, may not address the issue. |
Ban | Ban the use of AI tools in education. | Can deter cheating; | Difficult to enforce, students can bypass bans, may not be effective in the long term. |
Invigilate | Design assessments that circumvent the use of AI. E.g.: revert to traditional exam settings where students are monitored during exam. | Can deter cheating in traditional exam settings. | Not a widespread solution, resource-intensive, may not encourage learning. |
Embrace | Embrace GenAI in assessments. Could range from allowing students to use AI in specific tasks to having them critique, update, or assess AI-generated output. | Prepares students for future use of AI, encourages critical thinking. | Ethical concerns, varying student abilities in using AI. |
Design around | Design assessments around the limitations of AI. Exploit weaknesses of AI. | May work in the short-term. | With AI getting increasingly sophisticated, benefits will not last. |
Rethink | Rethink assessment entirely by asking how and why students are assessed in the first place. | Encourages creativity, aligns with learning processes. | Challenging to implement, not straightforward. |
The viability of each of these approaches over the short, medium, and long term can be represented by colors (red: likely not viable, orange: care needed, and green: seems most viable):
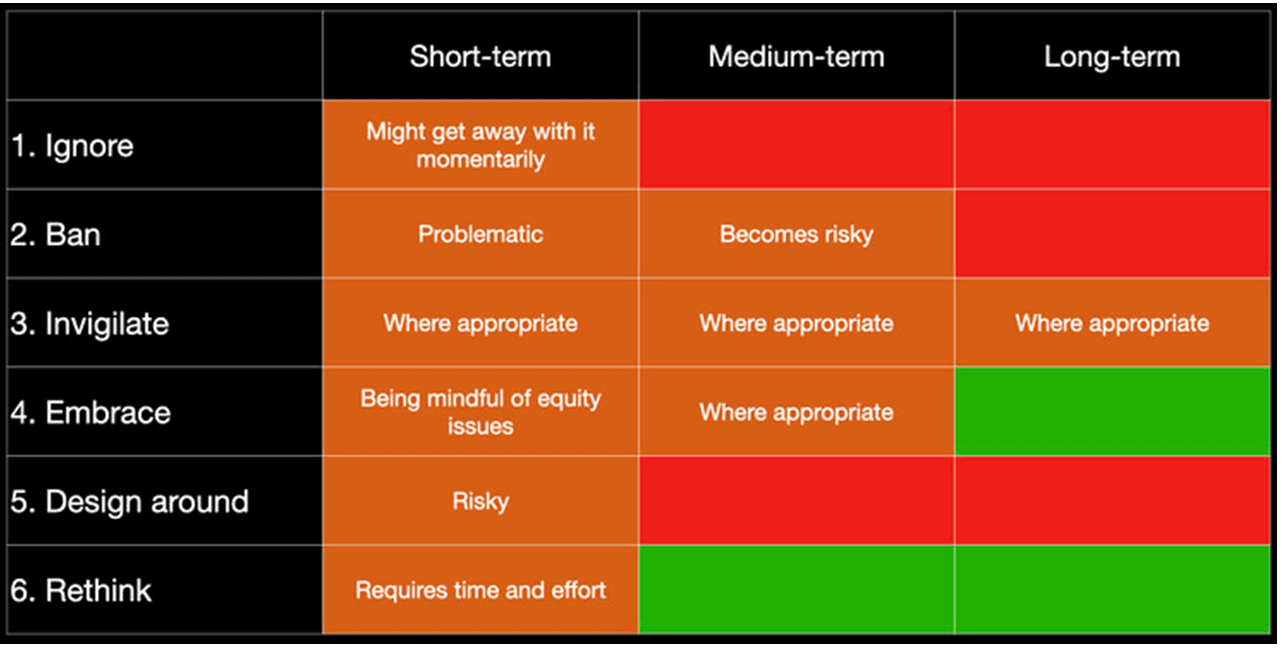
Each option presents complexities, and teachers will ultimately need to adopt a combination of approaches to address the challenges posed by GenAI. Rethinking assessment for the AI era will likely require coordinated efforts among researchers, educators, designers, learning technologists, and policymakers.
The ideal approach depends on the subject and content, but effective assessment should be authentic and reflect the skills students will need beyond the classroom. It should shift from mere knowledge verification to a focus on skill demonstration—including, where appropriate, the use of GenAI.